
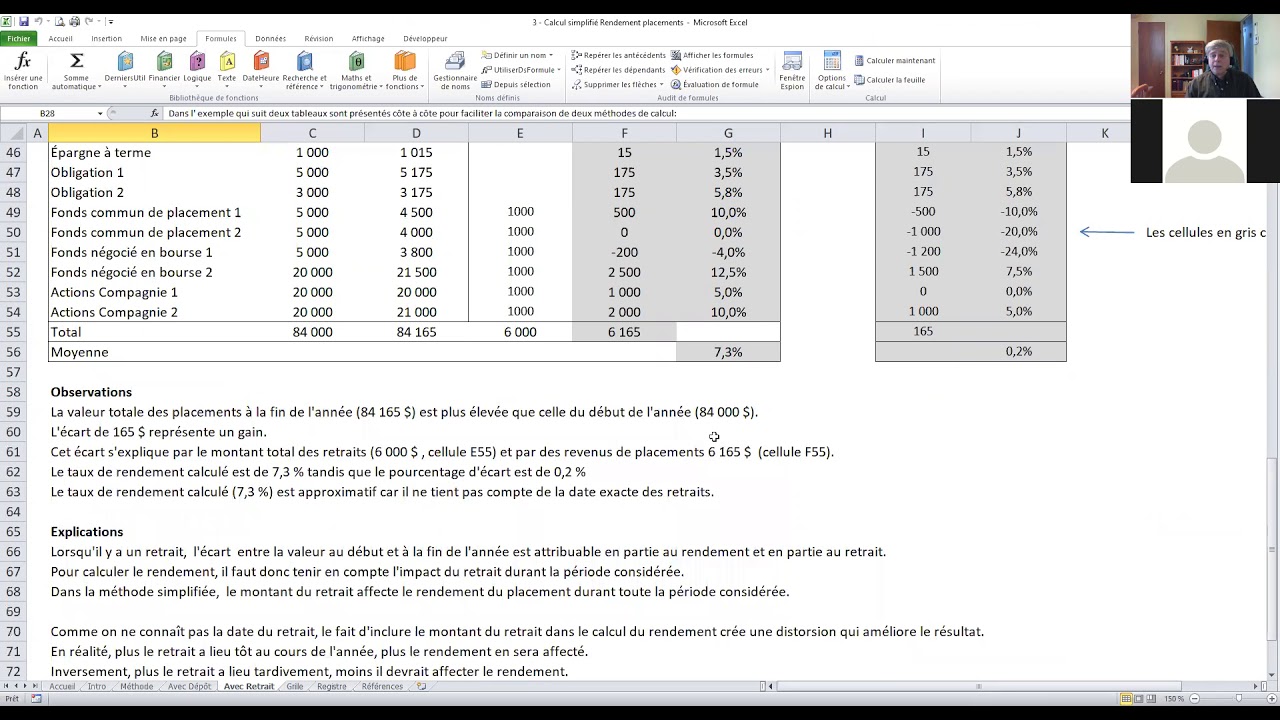
However, monitoring the crop canopy and production capacity of plants, especially for cereals, can be challenging. In semi-arid areas characterized by frequent drought events, there is often a strong need for an operational grain yield forecasting system, to help decision-makers with the planning of annual imports. Thus, the proposed simple light-use-efficiency model can be considered as a useful tool to correctly reproduce DM and GY values. For GY, R2, and RMSE values were respectively 0.71 and 0.44 t/ha for the developed approach and 0.88 and 0.37 t/ha for AquaCrop. Likewise, although of its simplicity, the accuracy of the proposed model seems to be comparable to that of the AquaCrop model. These correspond to a relative RMSE of about 19% for DM and 20% for GY. For the model validation, the obtained results showed a good agreement between the estimated and observed values with a Root Mean Square Error (RMSE) of about 1.07 and 0.57 t/ha for DM and GY, respectively. By contrast, a linear evolution was sufficient to represent the relationship between HI and CGDD. The model calibration allowed the parameterization of εmax in four periods according to the wheat phenological stages. Further, the outputs of the simple model were also evaluated against the AquaCrop model estimates.

The calibration and validation of the proposed model were performed based on the observations of wheat dry matter (DM) and grain yield (GY) which were collected on the R3 irrigated district of the Haouz plain (center of Morocco), during three agricultural seasons. The originality of the proposed method consists in (1) the modifying of the expression of the conversion coefficient (εconv) by integrating an appropriate stress threshold (ksconv) for triggering irrigation, (2) the substitution of the product of the two maximum coefficients of interception (εimax) and conversion (εconv_max) by a single parameter εmax, (3) the modeling of εmax as a function of the Cumulative Growing Degree Days (CGDD) since sowing date, and (4) the dynamic expression of the harvest index (HI) as a function of the CGDD and the final harvest index (HI0) depending on the maximum value of the Normalized Difference Vegetation Index (NDVI). The digital thread ties together a connected ecosystem for manufacturing and, with the addition of domain expertise and intelligence, enables continuous learning and improvement.In this study, a simple model, based on a light-use-efficiency model, was developed in order to estimate growth and yield of the irrigated winter wheat under semi-arid conditions. Our hardware solutions use metrology to bring real-world physical attributes to the digital thread to improve the accuracy of operations.
#Calcul rendement locatif belgique software
Together, our manufacturing intelligence software solutions create a digital thread throughout the manufacturing process, enabling the entire organisation to take a holistic approach and work together with speed and confidence to achieve the desired outcomes. Through an unparalleled portfolio of digital manufacturing technologies spanning CAE solutions for design and engineering, CAD CAM and complementary software for production applications, metrology hardware and software solutions, as well as data management and analytics tools, we empower technology users throughout the process with deep and actionable insight into product quality, ensuring that quality drives productivity.

Our manufacturing intelligence technology enables manufacturers to access, analyse and actively use data from all the key stages of the manufacturing process.
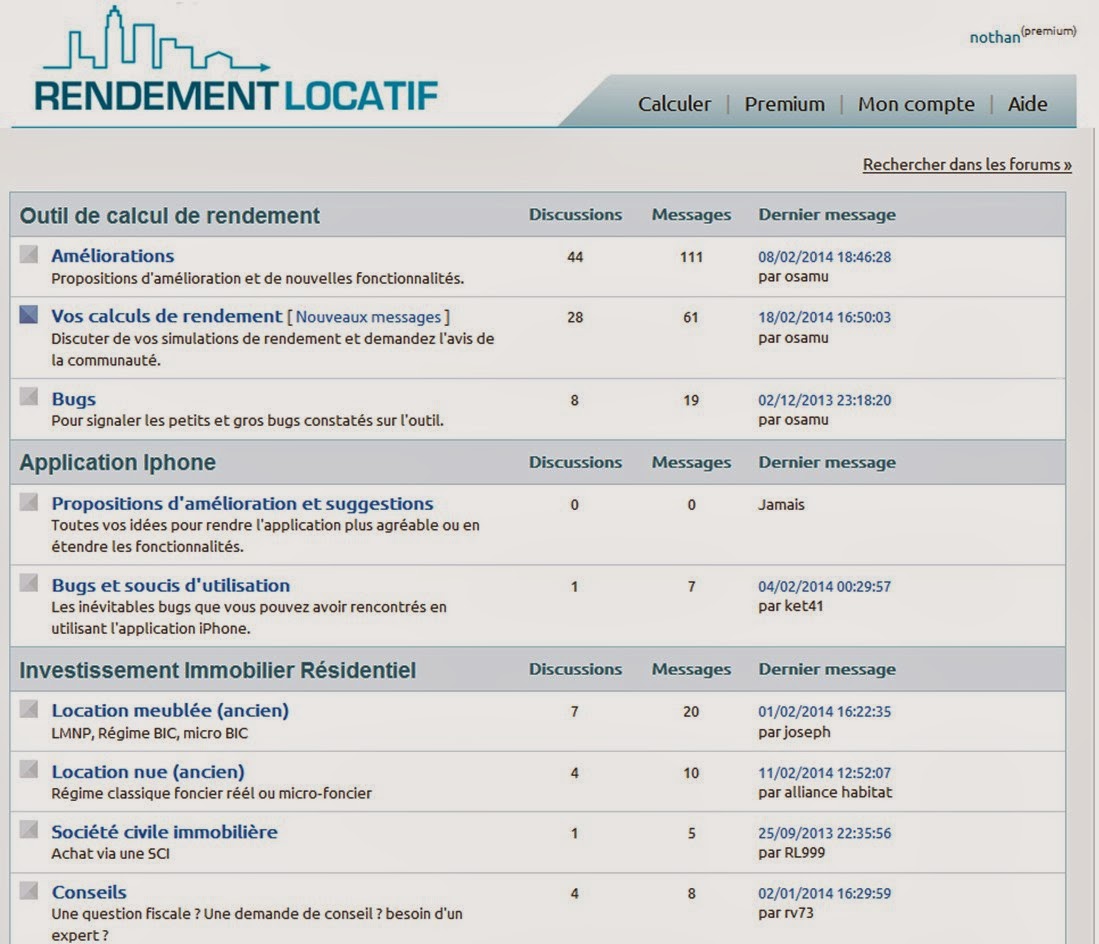
Hexagon's Manufacturing Intelligence division helps customers put data to work to improve productivity and efficiency while embedding quality throughout the product lifecycle. Continuously improving productivity is essential for competitive success. While specific industries have their own unique challenges and motivations, productivity is central to manufacturers across the board.
